Mathematician casts new models of uncertainty for a changing world
Ludovic Tangpi explores new ways to model and mitigate risks in large, ever-changing systems, with applications to financial markets.
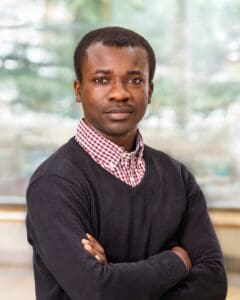
An assistant professor of operations research and financial engineering, Tangpi joined Princeton in 2018 after completing a Ph.D. at Humboldt University of Berlin and a postdoctoral fellowship at the University of Vienna. He is originally from Cameroon, where he earned a bachelor’s degree in mathematics at the University of Yaoundé 1. He also holds a master’s degree from a joint program of Stellenbosch University and the African Institute for Mathematical Sciences in South Africa.
In 2021, Tangpi received Princeton Engineering’s E. Lawrence Keyes, Jr./Emerson Electric Co. Faculty Advancement Award. He was also selected for a CAREER award from the National Science Foundation, the agency’s most prestigious award for early-career faculty members. His project, “A new form of propagation of chaos and its applications to large population games and risk management,” will develop a new framework for understanding complex interactions of agents in large populations. As part of the award, Tangpi is planning outreach activities to encourage more students from underrepresented groups to pursue engineering graduate school.
In recognition of Black History Month, Tangpi recently spoke about his early insight into the possibilities of mathematics, his research interests, and his plans for the CAREER award.
How did you get interested in mathematics?
For a long time in school, mathematics felt like a recipe. You had a given problem and you had to look into the recipe book and know what piece to apply. It was about memorizing tools to solve a given homework question.
But then, during my sophomore year of high school my teacher showed me something quite different. I understood that it was about putting together ideas in a logical way to achieve a given conclusion. That completely changed my perspective and made me want to know more.
As an undergrad, I studied mathematics not necessarily as a career, but mostly as something that I was curious about. When I started my master’s degree I began to wonder what to do with mathematics as a career, beyond working on problems and learning more, so the natural path was to be a researcher, because it’s all about learning and generating new ideas. That’s how I got into doing a Ph.D., and then it became a career for me.
What types of problems have you worked on?
When I did my master’s degree in Cape Town, I got interested in probability theory. I focused on studying stochastic processes and random events, and then dynamical systems, so I wanted to specialize in that area. In my Ph.D. work in Germany my focus was on backward stochastic differential equations and [their applications to financial risk management], and portfolio optimization. The specific problems were understanding how to optimally manage risk in dynamic settings. In fact, a financial agent having exposure to a future risky investment would typically like to be able to minimize (or manage) their risk not only at a specific date or time, but actually every single day, taking into account new market information to make new decisions. I worked on understanding how the concept of backward stochastic differential equations would help solve this problem.
That was a few years after the financial crisis [of 2008] which was partially caused by the fact that people were trusting models that were not completely accurate. Hence, the academic community was working on developing a theory of quantitative finance and risk management in a general setting where there are different models that can be correct. This is called model uncertainty because one is not certain which model is the right one. Of course, prices of contingent claims such as options and their hedging strategies are heavily influenced by the model that one uses, that is, picking two different models could lead to two different risk management strategies. Therefore, understanding this question is essential for the stability of financial markets. But it also leads to very interesting and exciting new mathematical questions and concepts such as a theory of stochastic calculus under several underlying probability measures, dual representations theorems in functional analysis or the concept of martingale optimal transport, to name a few.
I spent quite a bit of my Ph.D. and my postdoctoral fellowship working on these problems, both the underpinning mathematical theories and the financial market applications. Since I got to Princeton I’ve switched gears a little bit. In risk management, I’ve been working on more concrete problems — namely, understanding how to optimally simulate risk measures. We are trying to find efficient numerical simulations that are not model-specific, allowing us to simulate complex risk measures. For instance, based on historical data — you observe data on stock prices or option prices and use those data to simulate the risk that a trader incurs.
Have any of the frameworks you’ve developed helped to inform decisions in finance?
The work that we have done has allowed us to have more robust models that will help price financial derivatives differently. The outcome isn’t necessarily easy to adopt for practitioners because when you reduce risk it means that the prices become higher, and capital requirement constraints become more conservative, so banks should set aside more money to cover risk in this robust framework, and they have been reluctant to do that. The other difficulty is that even though mathematically we’ve been able to develop a nice theory, it’s hard to apply on the trading floors because these methods are sometimes hard to simulate numerically.
One aim of my CAREER award is precisely to address this issue by developing methods to simulate the solutions to some of these problems more efficiently, taking into account model uncertainty. This will bridge the gap between the theory that we have developed over the past 10 years or so and the more practical concerns that traders have. We are going to develop ideas from interacting particle systems, statistics and high dimensional probability to achieve that.
What other problems are you focusing on?
Another direction in my research has been understanding the behavior of interacting particle systems that evolve randomly either forward or backward over time. The general idea here is a setting whereby the behavior of one particle A influences another particle B, and if there are just a couple of particles then the problem can be easy to understand or to model, but difficulties occur when you have a very large number of particles. For instance, if you are looking at the portfolios of two traders it’s usually OK to analyze, but when you have a million traders it’s really hard to analyze and simulate their portfolios because of the high dimensionality of the problem.
The fundamental ideas from interacting particle systems are not new. When interacting particles are homogeneous or identical, they tend to converge to a microscopic model in which one can analyze a single representative particle. Think for instance of molecules in fluid dynamics. We are trying to replicate similar phenomena in the stock market, where we see each financial agent as one molecule in an interacting system, whose large population and long-term behavior we would like to understand.
What types of outreach activities are you planning?
When I look at the overall pipeline from high school to achieving a professional career in STEM [fields], it seems like we’ve been doing well in keeping students from underrepresented groups in school at the high school and undergrad level. The difficulty, it seems, is the transition from classes to research or from undergrad to graduate studies. Maybe these students do not necessarily know what graduate studies entail — what’s a Ph.D., how long it takes, how to apply and what type of questions researchers work on.
So, we’re going to try to help students who are graduating with a bachelor’s degree to stay in academia and pursue graduate studies. The outreach activities will include explaining what graduate school is, what research in mathematics or engineering is, and showcasing successful work that has been done by people from underrepresented groups. We also plan to host workshops for applications.
The outreach will also be about presenting our research as well as other works along the same lines to students, trying to explain it to a less specialized audience so that they’ll be motivated and understand how we can use mathematics to solve concrete and interesting problems. I believe that this will encourage students to be more curious about engineering applications of mathematics and hopefully try to pursue advanced careers in STEM.
For information on Princeton University resources and events for Black History Month, please visit https://www.princeton.edu/news/2022/02/04/imani-perrys-selections-black-history-month-plus-university-events-and-resources.