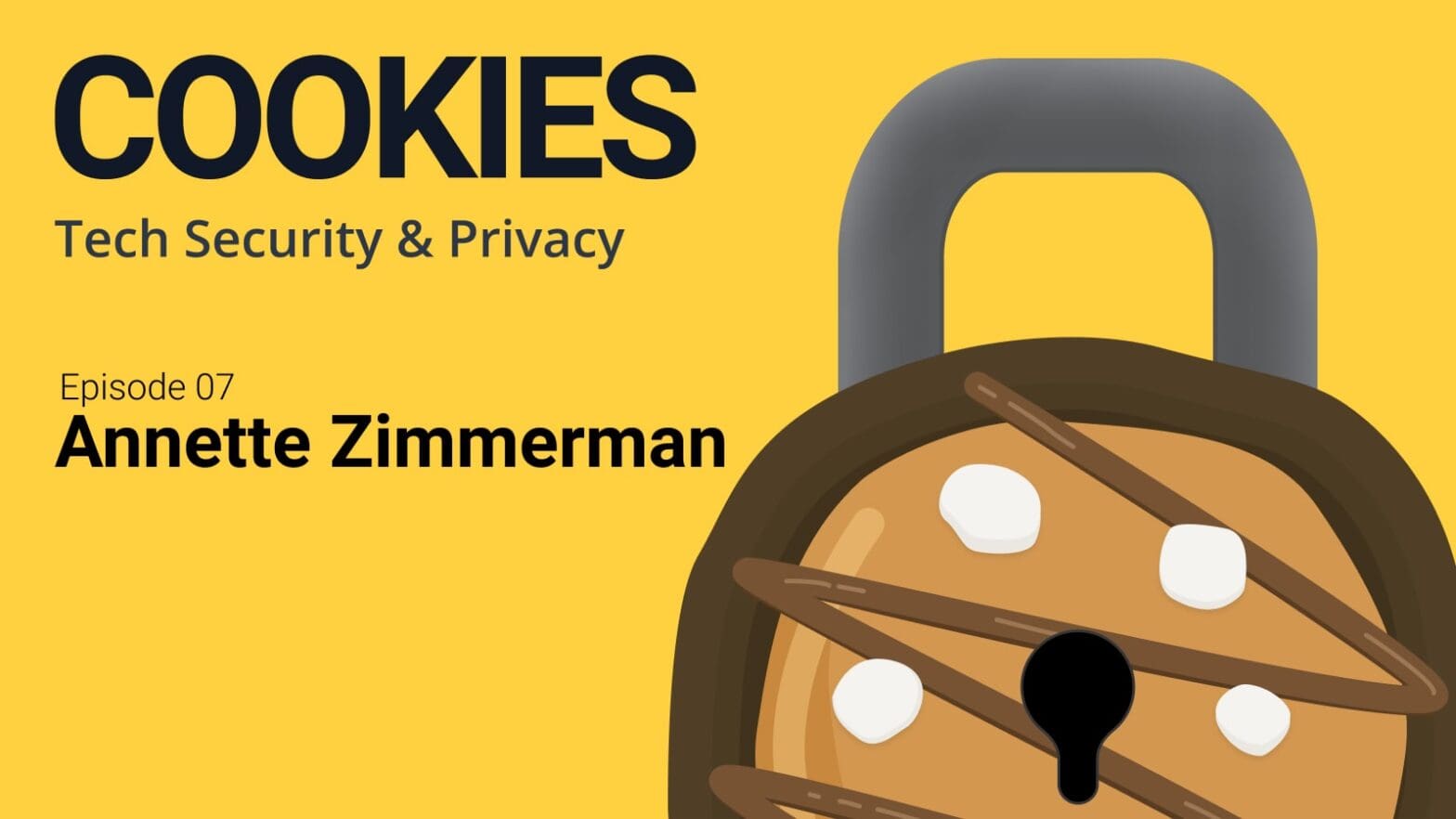
When It’s Best to Let A.I. Go Unused: Annette Zimmermann
By
on
This is from the series Cookies: Tech Security & Privacy, a podcast
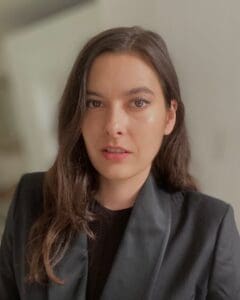
Annette Zimmermann makes the provocative argument that there are times it might be better to take cutting-edge artificial intelligence tools and leave them unused.
Annette is a political philosopher working on the ethics of artificial intelligence and machine learning. She’s a technology and human rights fellow at the Carr Center for Human Rights Policy at Harvard University, and an assistant professor in philosophy at the University of York in the United Kingdom. Annette was previously a postdoc at Princeton’s Center for Information Technology Policy as well as at Princeton’s University Center for Human Values.
Link: The research page of Annette Zimmermann’s website.
Transcript:
Aaron Nathans:
From the Princeton University School of Engineering and Applied Science, this is Cookies, a podcast about technology privacy and security. I’m Aaron Nathans. On this podcast, we’ll discuss how technology has transformed our lives, from the way we connect with each other, to the way we shop, work and consume entertainment. And we’ll discuss some of the hidden tradeoffs we make as we take advantage of these new tools. Cookies as you know can be a tasty snack, but they can also be something that takes your data.
Aaron Nathans:
On today’s episode, we’ll talk with Annette Zimmermann, who makes the provocative argument that there are times it might be better to take cutting-edge artificial intelligence tools and leave them unused. Annette is a political philosopher working on the ethics of artificial intelligence and machine learning. She’s a technology and human rights fellow at the Carr Center for Human Rights policy at Harvard University, and an assistant professor in philosophy at the University of York in the United Kingdom. Annette was previously a postdoc at Princeton’s Center for Information Technology Policy, as well as Princeton’s University Center for Human Values. Annette, welcome to the podcast.
Annette Zimmermann:
Thanks for having me, Aaron.
Aaron Nathans:
All right. So first, in a recent article you said, “Data is not just a social mirror, it’s a magnifying glass.” What do you mean by that?
Annette Zimmermann:
It’s really common for people to say something like, “Well, garbage in garbage out.” So our technological models are only as good as the data that we put in them. And that much is true. In fact, we might see this kind of phenomenon as actually an indicator of good design in a lot of ways. So one key design goal is to make sure that our technological models give us a faithful reflection of social reality. So computer scientists of course, call that calibration. And so when we have well calibrated models, what we see is they’ll reflect social reality pretty well, including all of the social disparities that we have in society. So, that’s a problem for us, because it’s not obvious how exactly we should intervene in order to fix that. One phenomenon in this context that I think is particularly important, is this human phenomenon of automation bias. So, we know empirically that humans are really much less likely to question a decision making output, given an outcome that results from a process that we view as quantifiable and ostensibly neutral and objective and data driven.
Annette Zimmermann:
We’re just much less likely to intervene critically in that kind of decision making process and question, whatever assumptions might have underpinned that process. That is dangerous, because it might prevent us from intervening where normally we would be intervening, right? So contrast an algorithmic decision making process to a human decision making process on some type of issue, let’s say we’re predicting something like criminal recidivism risk. Now obviously, human decision makers are highly biased. So that’s a major problem, that’s why we move towards automation in this area in the first place. But then of course, it’s not strictly true that automated decision making in the domain of criminal justice is completely bias-free. Very often, we find highly disparate distributions of error rates across different socio demographic groups in that area.
Annette Zimmermann:
Now, the compounding problem here is that once we have that tool that works imperfectly, we have this additional issue that humans interacting with that technological model and making decisions based on those algorithmic outputs are much less likely to ask this question of what was the purpose of automating this in the first place? And what do we not know when we are confronted with this output? Right? So what can we do with technology and what can’t we do? Now, this can lead to a process of amplifying social disparities, right? If we leave technology unchecked, then it’s much more likely that they will scale up existing disparities further and further in a way that is unfettered by normal processes of human deliberation and critique. Now, in addition to magnifying those social inequalities, technology can also simultaneously obfuscate them. So it’s quite a tricky, pernicious problem.
Annette Zimmermann:
So on the one hand, it’s possible that over time if we leave technology unchecked and don’t intervene to make it more accurate and more fair, social disparities can turn into much larger cleavages, but also, the mere use of an algorithmic tool might endow these decision making processes with a false sense of certainty or accuracy. And so it can thereby obfuscate the degree to which those systems actually spin out from our control. So, there’s a kind of double problem space here that isn’t quite captured by this very neat thesis that data just reflects social reality, it’s actually a much bigger problem than that.
Aaron Nathans:
So why do people tend to defer to data? Why do we get the impression that we’re taking our biases out of the equation, that the numbers speak for themselves?
Annette Zimmermann:
Well, I think it’s natural to think that as soon as we work with more fine-grained information, we’re going to get better quality decision outcomes, right? So very often, the move to automate a given decision task is driven by this underlying assumption that we’re just working with really imperfect information. And obviously, our own human reasoning processes can be flawed, they can be irrational, they can be biased in multiple ways. And so if that’s true, the assumption is, well, one good way of solving this problem is just getting more data and getting better decision making processes in the mix. Now, the tradeoff we’re making here is, on the one hand, we’re using much more fine-grained data, and we can compute particular problems at an unprecedented scale, but we’re also reducing our amount for leeway in decision making, right?
Annette Zimmermann:
So given this problem that we just talked about, this problem of automation bias, it becomes harder and harder for us to recognize that as human decision makers, we can’t adopt this kind of attitude of helplessness, right? We can’t just outsource all of the decision making process through technology, we have to keep thinking about what our role is when we interact with technological models. So, one good thing that I think we ought to do as human decision makers routinely whenever we interact with technology, is to intervene early and often. What that means is that we start thinking about the stakes of using automation in a given domain as soon as we start the design process. So we shouldn’t view ethical reasoning or political reasoning, as a kind of final checkbox towards the end of the design process. No. That needs to start much, much earlier. So we need to ask ourselves these really complex questions about well, what’s the purpose?
Annette Zimmermann:
What’s the goal of automation in this domain? So do we really need to predict this particular thing? Do we… Maybe you want to predict some other thing. And so answering these kinds of questions will really inform the shape that a design process can take. In addition to that, we also have to recognize the importance of changing our minds down the line. So, it might very well be true that initially it seems like one particular decision problem really lends itself to automation. So there’s a really strong presumption in favor of optimization and innovation in that area. And that’s a really good thing. But we shouldn’t get trapped in a path dependency here, right? So we should remain open to the idea that at some point it turns out that actually down the line we might choose to not deploy that tool anymore, and instead develop a different tool instead, right? In light of new empirical information that we learn when we see how the model interacts with the social world.
Annette Zimmermann:
So for instance, under conditions of severe social inequality, using a particular tool that makes certain social processes run more smoothly, might actually undermine justice. So just to give you a concrete example here, think about any sort of AI-driven application in law enforcement and criminal justice. So, if we’re operating under social conditions that are really systemically unjust, right? Like in a way where it’s really unclear if technology alone can fix those social problems. It’s plausible in my view to think that very often technology alone is insufficient for addressing these problems. We also need much larger-scale political and institutional transformations. But if we’re operating under this reasonably unjust status quo, then using a tool that just accelerates what we currently have that just scales up those disparities, will actually not be the best thing we can do here, right? So whenever we automate, we have to be cautious that we’re asking the right kinds of questions about the purpose of scaling up a given social practice or social phenomenon.
Aaron Nathans:
I can’t tell you the number of times I’ve heard… “If history is any guide…” Is there a downside when statistical models use past performance as a guide for future decision making? What’s a good example of using old numbers to perpetuate old problems?
Annette Zimmermann:
I think there have been a lot of good recent examples in many different domains of this kind of phenomenon. So, think for instance of this recent scandal in relation to Amazon’s algorithmic hiring process. So, Amazon had this tool that ranked different applicants for a given position. And this tool placed a really high premium on hiring for a company fit. Right? So the idea was, “Well, our teams currently work really well, we want to attract applicants that fit in that team and kind of slot right in.” But what the tool did was it systematically downgraded female applicants because women were really underrepresented in that current team. And so of course, this is an instance of bad design rather than explicit malice. But the effect was nevertheless, really severely unjust, right? Because if you had something like a woman’s soccer team captain on your CV, you were automatically downgraded by that algorithm just because there was an underrepresentation of female employees in that team.
Annette Zimmermann:
So, here learning from past data was done badly. That doesn’t necessarily show that learning from past data is always objectionable, but it can be if we don’t think carefully about just exactly how we want to measure a variable like hiring for a company fit, right? So we might have to adopt a different view on what a good applicant actually looks like. And so measuring and operationalizing that concept isn’t straightforward, right? So we have to ask these deeper questions here before we just base our decisions on what we’ve done in the past. Another really good example arises in various healthcare applications of AI. So, there have been some recent studies that show that very often algorithmic decision making used in diagnostic contexts disadvantages Black patients. And the reason was that fairly blunt and reductive algorithmic tools were being used for these diagnostic purposes. And in particular, those tools really focused on past data on the cost of various health care interventions.
Annette Zimmermann:
And of course, what skews this data, this past data pattern, was that historically, Black patients have received less care for really serious conditions, right? Like less costly care for many different kind of systemic reasons. And so inferring future treatments, future medical treatments based on these skewed data sets from the past, can just perpetuate these racial disparities that we see in the healthcare sector. So, that’s another good example that really shows that we need to think very carefully about just what kind of past data we would like to base our future decisions on. Very often the concepts that underpin algorithmic designs are inherently contested. Right? They’re not straightforward concepts. The same is true in these criminal justice applications that I mentioned to you before. So, whenever we’re working with a system that tries to classify people in categories like high-risk or low-risk, we have to ask this question, well, is it obvious how we should measure riskiness on an individual basis?
Annette Zimmermann:
As it turns out, that’s a really politically and ethically contested category, right? Which variables should we use? Should we just base this on prior arrest data? Well, if we do, we’re going to run into this problem that arrest records don’t neatly map onto crime rates, right? Especially when we have biased policing practices, we’ll see that a lot of people get arrested who actually aren’t guilty of a crime. But conversely, we might not arrest some people who actually are criminals. And so often, we’re working with really imperfect measurements here for what we’re actually trying to predict and classify.
Aaron Nathans:
You write in an upcoming paper about deciding who deserves the benefit of the doubt when it comes to measuring a student’s potential using artificial intelligence. What did you mean by that?
Annette Zimmermann:
In this paper, I’m commenting on a recent political controversy in the U.K. So, during the COVID-19 pandemic, the U.K. government decided to just cancel all final high school exams, and instead, replace them with this algorithmic prediction tool. Now, this is a really important issue because in the U.K., final high school grades really have a huge impact on people’s lives. So, they determine things like university admissions, and there was an uproar initially, because people were really concerned that using an algorithmic tool of this kind might actually disadvantage students who go to schools that have been performing more poorly in comparison to other types of schools. Now, it’s relevant to have background knowledge in this context that the U.K. school and education system is really stratified around socio-demographic variables like race and class. So that was already at the forefront of people’s minds when this controversy arose. And lo and behold, when the algorithm was implemented, it actually did end up downgrading people, especially students of color and working class students.
Annette Zimmermann:
About 40 percent of students’ grades were downgraded. And not only just from an A to a B, it was more like, you might go from an A to a fail. So there were really significant disparities in terms of these outcomes. So as it transpired, one reason why this algorithmic tool worked so badly, was because it didn’t really include a good way of measuring uncertainty. So for instance, it made these historical predictions for schools that were rapidly improving over time. So there were a number of schools in the U.K. who performed really badly initially, but then scaled up and really enhanced the quality of education. And in those schools, the data was really mixed, right? So, up until five, four, three years ago, results were really bad, but then students who deserved really good final grades… actually, would have gotten those grades if they had sat exams, didn’t receive them. So many of those kinds of students failed. And so the reason here was that the tool didn’t have a good way of putting into context that that school had improved rapidly over time.
Annette Zimmermann:
Now, being able to factor in something like our confidence or degree of confidence in a particular result given recent historical changes, that would have been the thing that made that algorithm better. But the algorithm was fairly crude. And so it wasn’t able to put these complex social phenomena into your critical context. And of course, again, because of these empirical conditions like automation bias, not many other people were questioning this tool either. So the algorithm itself canceled this problem and human decision makers opted out of questioning the tool. Now, this combination of bad factors, led to really, really unjust outcomes to the point where teenagers were protesting in the streets. To my knowledge, that’s the first in-person protest against algorithmic injustice. So I thought that was a really interesting phenomenon, and I think we’re going to see much more of that going forward.
Annette Zimmermann:
And so what these students said was, “Well, I feel like I’ve been slotted into the category of people who just never had a shot. I’m not being given the chance to prove myself, either in an exam context or while being assessed algorithmically. I’m just already slotted into this category of somebody who can’t win.” And so that’s what made that tool really unjust. People felt like they weren’t being given the opportunity to really excel, because they were from schools that have historically performed poorly.
Aaron Nathans:
So it’s broader than that though. AI sometimes just keeps people from having the benefit of the doubt all across our society, right?
Annette Zimmermann:
Absolutely. So, exactly this kind of phenomenon occurs in many other domains. And so it’s an interesting phenomenon, I think, because a lot of people might look at AI and kind of think of AI as this fundamentally uncertain or inscrutable black boxy type of thing. So… Now, of course it’s true that machine learning requires some degree of opacity. In fact, some of our most sophisticated machine learning tools work especially well because they allow for some degree of opacity. But that doesn’t mean that algorithmic systems don’t also reduce uncertainty in a lot of ways. As soon as we measure and quantify something, we’re already making choices about what is worth measuring, and how confident should we be about the predictions that we make based on those measurements. And so that’s the area where things can get very tricky. But on the one hand, we think, well, numbers are neutral, but on the other hand, I think we have to realize that technology never operates in a moral and political vacuum. Right?
Annette Zimmermann:
It’s not like we can do technological design in a completely value-neutral, value-free space, we’re already making at least implicit judgment calls about what matters and how best to measure what matters. And that’s exactly where these problems come in. And we can find it in AI applications and education, but also criminal justice, hiring, credit scoring, all of these other kinds of decision problems where we use automation to make our lives easier.
Aaron Nathans:
So, there’re some really weird AI out there. And you’ve written about this. What are some examples and what do you think that tells us about how people are choosing to use technology?
Annette Zimmermann:
Right. I think recently, there have been a number of AI applications that seem to work relatively well. So, they do kind of well with respect to accuracy. But their purpose itself is badly defined, right? So for instance, consider this recent wave of beauty assessment tools. So there’s a number of facial recognition systems that give users a beauty score. And so basically, the way in which those tools work is they just measure certain distances between various facial features, and then they tell you how symmetrical your face is. My question when I look at these tools is always, why do we need that? So the companies pitching these kinds of tools have these really amazing websites where they say something like, “Measuring beauty is really hard, but we’ve developed this way of doing it.”
Annette Zimmermann:
But again, that doesn’t really answer the question of why we need an automated way of determining facial symmetry, and how that moves us further as a society. So, on the one hand, it reinforces this assumption that symmetrical faces are just inherently more desirable, that there is some objective standard of beauty which I think we have strong ethical and political reasons to question. But then also, and even more perniciously, it transpired that those tools again exhibited a lot of racial bias. So, one of these tools just completely eliminated dark-skinned faces from its ranking of the most beautiful people it had assessed. And in a way that’s predictable, because we’ve seen similar racial bias problems in many other applications of AI. Often the problem is, well, developers didn’t really think carefully about what the standards that they want to impose on their systems should be, which data sets are being used and so on.
Annette Zimmermann:
But just because that problem is predictable, doesn’t mean we should adopt this attitude of leaning back and just kind of saying, “Oh, all AI kind of scales up these disparities.” No. We should question the purpose of using that tool in the first place. It’s also worth noting in this context, actually, that even if one type of AI tool just seems weird in the sense that its purpose isn’t super clear, that doesn’t necessarily make it laughable or kind of innocent. So we might look at this beauty assessment tool and kind of think, “Well, that’s kind of an unnecessary and potentially misguided tool.” But we can opt out of using it. Nobody’s forcing you to get a beauty score from this AI tool. But the same companies that develop these kinds of technologies often also use their expertise in this domain of facial recognition for much more nefarious purposes. So, recently for instance, it was revealed that one of these beauty scoring tools called Face++, which was developed by a Chinese company called Megvii.
Annette Zimmermann:
That kind of tool enabled Megvii to then collaborate with Huawei to test a tool called Uighur alarm. Now of course, the purpose of this tool was to use facial recognition to identify and profile and potentially arrest members of the Uighur minority and China. And so we can quickly see that weird AI can turn into a very bad, explicitly bad, explicitly discriminatory AI application very quickly, because the same kind of inference method can be used for many different kinds of purposes. So, we shouldn’t look at weird AI with complacency, I think we should always question what’s the purpose of this tool and how could that purpose be redefined into something that is even more dangerous? So it’s important to kind of ask these deeper questions about these kinds of applications, I think.
Annette Zimmermann:
Now in addition, maybe just another example that I found interesting, this came out a few months ago. Amazon has a new health band called Halo. And this is a so-called holistic health tool that aims to assess not only your physical health, but also your mental and emotional well-being. What you do when you get it is you do a near naked body scan with it in order to assess your BMI. Of course, all of that data gets sent to Amazon straight away. I had immediate worries about that aspect. Of course, not only do we know that body mass index is really bad measurement of physical health. But I was also very concerned about the privacy implications of sending a lot of this kind of data to a company like Amazon. But that didn’t stop there. So this tool as I mentioned, was aimed to be more holistic, which in my view is a euphemism for just trying to gather even much more data. So one thing that the tool does is it listens to you all day, and it evaluates the tone of your voice.
Annette Zimmermann:
Now, emotion recognition is notoriously controversial in the fair machine learning community for good reason. People are skeptical of this idea that we can really accurately measure emotions based on fairly blunt metrics. Now, people who tested this tool found that it worked really differently for different people. So a team of journalists recently, a male and a female journalist wore it for a day. For the male journalist, it came out with things like, “Oh, you sound very assertive today. You seem to be doing great.” Unsurprisingly, the female journalist got very different feedback. It was something like, “Oh, you sound a little opinionated there.” So, it was the high-powered equivalent of being told to smile more. So we might question whether that’s actually conducive to health assessment, holistic or not.
Annette Zimmermann:
And so I think the purpose of this kind of tool just clearly hasn’t been thought through. It’s not only that it’s invasive, even though of course, that’s a major, major problem here. It’s also just unclear why we need this kind of invasive data collection. And if the benefits of doing that are really that clear. So asking this deeper question again, what’s the purpose of using AI in this domain? I think it’s really, really crucial.
Aaron Nathans:
How much is facial recognition being used in policing? And is it regulated?
Annette Zimmermann:
Right, that’s another example of a potentially weird and bad application of AI in my view. Recently, a team of Stanford researchers developed this really controversial tool that aimed to predict personal characteristics, behaviors, and preferences, again, purely from blunt facial biometric data. So, things like measuring the distance between your eyes. Now, the tool didn’t only predict things like your propensity for violent criminality based on that eye distance and other kind of facial features, it also predicted things like, are you likely to be gay? And I found that very puzzling. Again, I think the natural question, the first kind of question we should ask is, why do we need AI to determine whether people are gay based on the way in which their face looks? And again, why do we need to make this inference about future criminality based on the way you look? It kind of buys into this idea that your face is your destiny, which is a really flawed historical idea.
Annette Zimmermann:
So the use of this kind of tool in my mind, really echoes, really flawed inferential methods. For instance, methods used by eugenicists, or phonologists. So again, in the past we see these instances of pseudo researchers using fairly blunt assessments of people’s faces and skulls and making really far reaching predictions about their character, their behaviors, the likelihood that they’ll do certain things. And so even if the new kinds of tools that we have, something like the Stanford tool, which according to the researchers who developed it worked really accurately. So even if those kinds of tools now work well, I think we can’t really dismiss this worry about the historical baggage that is associated with these tools. Philosophers call this kind of problem expressive harm. So the mere use of a particular type of method that echoes a history of racist pseudoscience could be objectionable even if the researchers’ data suggests that the tool was actually able to predict who’s gay or, it was able to predict who’s a criminal.
Annette Zimmermann:
The kind of communicative downside of using this tool of choosing to use automation for this purpose entrenches this flawed idea that it’s true that some people just look gay or look like they’re criminals. And that’s bad, even if we can get the tool up to a standard that maybe outperforms humans making similar predictions. Now, you also asked about whether facial recognition technology in policing is regulated. It’s not very much regulated, but I think more regulation in this area is coming. We’ve seen some instances of members of the public, really contesting the use of facial recognition tools in law enforcement and immigration enforcement. So multiple American cities for example, like San Francisco, or Somerville, have locally decided to ban the use of these tools for policing purposes. So, San Francisco for instance, passed the stop secret surveillance ordinance. And the explicit reason cited in that ordinance for banning this kind of tool, was again, the racially disparate impact of that tool.
Annette Zimmermann:
So the idea here was, well, under current social conditions, we just can’t use this tool in a way that doesn’t scale up systemic inequality. So then our only option in this instance, is to ban it until we are operating under changed empirical conditions. And the interesting thing here is that not only that activists and policymakers come to this conclusion, major tech corporations also came to the same conclusion. So last summer, multiple companies like Amazon, and IBM, all put out these public statements that they were voluntarily imposing these moratoria on themselves. So they were saying, “We will not deploy this technology in this domain anymore, until this domain of law enforcement is properly regulated.” So even they were relatively nervous about empirical findings that showed that these tools failed really differently for white people than for black people and for men in comparison to women. So given that we know that when those tools go wrong, they go wrong in drastically worse ways for marginalized communities.
Annette Zimmermann:
The kind of result of that was that a lot of players in the tech industry and beyond responded by becoming much more friendly to this idea of at least temporary non deployment. Now, of course, I think the question here is, well, are we happy with just local bans, or do we want kind of industry wide regulation across the board? I think the latter option would be much preferable. So it would be a bad state of affairs to have really patchy regulation in a high-stakes domain like law enforcement and criminal justice. Because if you’re a citizen living in a democratic society, you want your rights to be secure in exactly the same way in every location. And so having really disparate policymaking on this issue, would undermine a really fundamental democratic value, namely the equal enforcement of people’s rights, no matter where they are.
Aaron Nathans:
You’re listening to Cookies, a podcast about technology security and privacy. We’re speaking with Annette Zimmermann, technology and human rights fellow at the Carr Center for Human Rights Policy at Harvard University, and an assistant professor in philosophy at the University of York. It’s the 100th anniversary of Princeton’s School of Engineering and Applied Science. To celebrate we’re providing 100 facts about our past, our present, and our future, including some quiz questions to test your knowledge about the people, places and discoveries who have made us who we are. Join the conversation by following us on Instagram @EPrinceton. That’s the letter E, Princeton. But for now, back to our conversation with an Annette Zimmermann. What is an example of a machine learning system that should not be deployed in the first place?
Annette Zimmermann:
Yeah, that’s a really controversial question, I think ,because a lot of people particularly in the tech industry, I think for good reason, think that whenever we can optimize something and build something that might be useful, we should, right? We should try it. And so the idea of simply not building something that we could in fact build or not optimizing something that we’ve already built, that’s just like giving up to a lot of people, right? And it’s maybe counterintuitive to say something like don’t build this tool or don’t deploy this tool. Because it sounds kind of like you’re trying to actively just not make things better. And so that’s what’s counterintuitive about it. Now, we can see this controversy play out exactly with the earlier example that we’ve talked about, namely facial recognition and policing. So here’s an interesting process that happened after local band were implemented in multiple cities, and certain companies self-imposed these voluntary deployment moratoria.
Annette Zimmermann:
Not everyone agreed that non-deployment was the best option here, so some companies said, “No, listen, optimization would benefit everyone in society. So even if we currently have disparate distributions of error rates in these tools across different socio-demographic groups, if we can just make these tools better, then that will move us closer to justice.” And so in particular, Google had an analysis of the problem space here, which was basically that the reason why we had these disparate distributions of error rates was because the data was just under-representative of society at large. So their problem analysis was, “Well, we need to make sure that our database has enough dark-skinned faces in it.” Now, the way they went about solving this problem was deeply objectionable in my mind. What they did was they employed a subcontractor, and that subcontractor decided to explicitly target and approach Black and homeless people in American cities like L.A. and Atlanta. And in order to incentivize them to provide this biometric data for optimization purposes, they offered these people a five-dollar gift card, like a voucher.
Annette Zimmermann:
And the conversation that unfolded in these exchanges, as employees of that subcontractor later on reported to the media, was something like, “Hey, here’s a selfie game, why don’t you play this game for us and we’ll just give you this voucher?” At no point was it mentioned that really fine-grained biometric data was being recorded from these people. Another variation was something like, “Just complete the survey, here’s a phone, you just kind of tick some boxes and do it really fast. Don’t think about a too much. Just do it really intuitively.” So employees of the subcontractor were really explicitly instructed to rush people through the process. And when people said something like, “Oh, is this recording my face right now? It looks like it’s recording.” They were told to say something like, “Oh, don’t worry about that, and no, it’s not.” And so that was really morally and politically objectionable from my perspective for multiple reasons. The first reason is that it’s incredibly deceptive, right? So it’s gathering a lot of invasive data from people by deceiving them into providing this information that they might otherwise not necessarily provide.
Annette Zimmermann:
And mind you for relatively small financial incentive. So, it looks like a kind of clear cut case of exploitation. Now, of course, some exploitative arrangements can be mutually beneficial. Political philosophers have analyzed those types of scenarios extensively. So, you might look at this kind of case and say, “Well, Google and the subcontractor stand to gain massively, financially, from optimizing facial recognition technology by using this data, and they’re paying people a mere five dollars for it, it looks like they’re taking an unfair advantage of them.” But, exactly those populations that are being exploited might ultimately benefit from these more accurate tools. Now, I think that’s a bad analysis because it doesn’t really show that exploitation in this context isn’t objectionable. But I think it’s still wrongful to deceive people in this way and to obtain this data in this very hidden and sneaky way.
Annette Zimmermann:
Now, in addition, I think the problem is that this optimization effort, which was posited as a real alternative to just not deploying these tools, that optimization effort itself undermined justice. It undermined justice because it exploited exactly the kinds of marginalized communities that were previously already burdened by badly functioning tools deployed in this area. So, it was almost like this optimization effort was adding insult to injury. And I think that compounds the initial wrongness of having a really racially biased facial recognition tool used in policing. Now, this kind of case in my mind really shows that unless we find a better way to optimize these tools, and unless we can implement larger scale social and political changes that make policing and criminal justice better and more equitable, then we should indeed not deploy these tools, right? We should temporarily say, “No, we’re not there yet. We actually can’t use technology in quite this way under current empirical conditions, so we need to make some other maybe unrelated-to-AI type changes before we can automate in quite this way.”
Annette Zimmermann:
Of course, this is subject to change. So if we’re successful in making society more just, then it’s very possible that we can use automation in multiple ways and multiple domains. But I think it’s important to recognize that in cases that… (inaudible) the structure of the case that I just explained, we shouldn’t shy away from something like non deployment, right? So even if there was a strong initial presumption of optimization, we should make some exceptions to that when we see that the results are just going to entrench injustice even further.
Aaron Nathans:
How do we make sure that as we build AI that we’re doing it for the right reasons and using it in ways to build a more just society?
Annette Zimmermann:
Right. I think the first thing we need to do is to really start valuing uncertainty in our collective decision making about powerful technology. So we’ve talked before about how often the appeal of AI seems to be that it kind of helpfully reduces uncertainty for us. It kind of condenses our decision making to issues that we can easily quantify and measure. And there’s something reassuring about that. But on the other hand, I think it’s always important to remember what we don’t know when we make quantified decisions, right? So welcoming this idea that some things might not be easily quantifiable. Sometimes we might need to argue and deliberate as flawed human decision makers that we are about how best to measure something, how best to reach a decision in a given context.
Annette Zimmermann:
And so allowing for this productive type of uncertainty and skepticism and doubt, that I think will really positively contribute to collective conversations about what AI should and shouldn’t do. Now, in order to do that well, I think those conversations shouldn’t be confined to the tech industry and to academia, I would welcome a development where there are more, broader kind of social deliberations on this issue. What we’re currently seeing something that we might call AI exceptionalism, this idea that AI is really unique, and really powerful, and really inscrutable and just really complex so that ordinary citizens can’t possibly dream of understanding it. Now, that’s true in the sense that ordinary citizens might not be tech developers, right? They might not be able to actually design these tools, but we can make value-based decisions about the purpose of using automation in various areas, much like we make value based-decisions about really complex environmental policy decisions, or other science and technology policy decisions, medical policy decisions.
Annette Zimmermann:
And so we should recognize that we as a democratic constituency, are actually already in the habit of deliberating about really complicated things. Often, that isn’t perfect, which is why we consult experts. But it’s not like we should simply opt out of scrutinizing the ethical and political implications of using AI completely. It’s very often we can say something about, does this tool really promote justice in this area, or should we rather just put ourselves through a slower human decision process here? Just to make sure that we can keep things in check there. And so often, those kinds of conversations I think, are going to be insightful. So we shouldn’t outsource this responsibility, the responsibility to deliberate critically on AI, just to corporations and experts. Now, at the same time, I think we should also seriously consider how decision making processes within the tech industry could be productively changed. Recently, a lot of tech employees and big corporations have voiced discontent with the ways in which decision making structures work in their companies.
Annette Zimmermann:
So this idea that often lower-level developer teams or individual developers don’t feel like they can pump the brakes on a given decision to build and deploy a tool. So if you foresee problems as an individual tech employee, do you really have meaningful opportunities to critically contest a kind of company-wide design process? And so allowing people within those corporate structures to exercise their critical judgment without fear of repercussions is really important, but also increasingly under threat. Recently, we’ve seen a number of high-profile firings of really important experts working in this area, I’m thinking in particular of Dr. Timnit Gebru, being fired from Google. Of course, Dr. Gebru was the co-lead of the ethical AI team there. So this kind of example, I think, really highlights how important it is to think about how individual employees in tech companies can really exercise their voice and their kind of critical input when it comes to thinking about whether AI should be used for various purposes. So, that’s a good reminder of where things currently work not well, and where there is significant room for improvement.
Aaron Nathans:
Well, thank you. This has been fascinating. This is a lot of great food for thought. Thank you, Annette.
Annette Zimmermann:
Thank you for the great conversation.
Aaron Nathans:
We’ve been speaking with Annette Zimmermann, a technology and human rights fellow at the Carr Center for Human Rights Policy at Harvard University, and an assistant professor in philosophy at the University of York. I want to thank Annette as well as a recording engineer Dan Kearns. Cookies is a production of the Princeton University School of Engineering and Applied Science. This podcast is available on iTunes, Spotify, Stitcher, and other platforms. Show notes and an audio recording of this podcast are available at our website, engineering.princeton.edu. If you get a chance, please leave a review, it helps. Views expressed on this podcast do not necessarily reflect those of Princeton University. I’m Aaron Nathans digital media editor at Princeton Engineering. Watch your feed for another episode of Cookies soon. Peace.