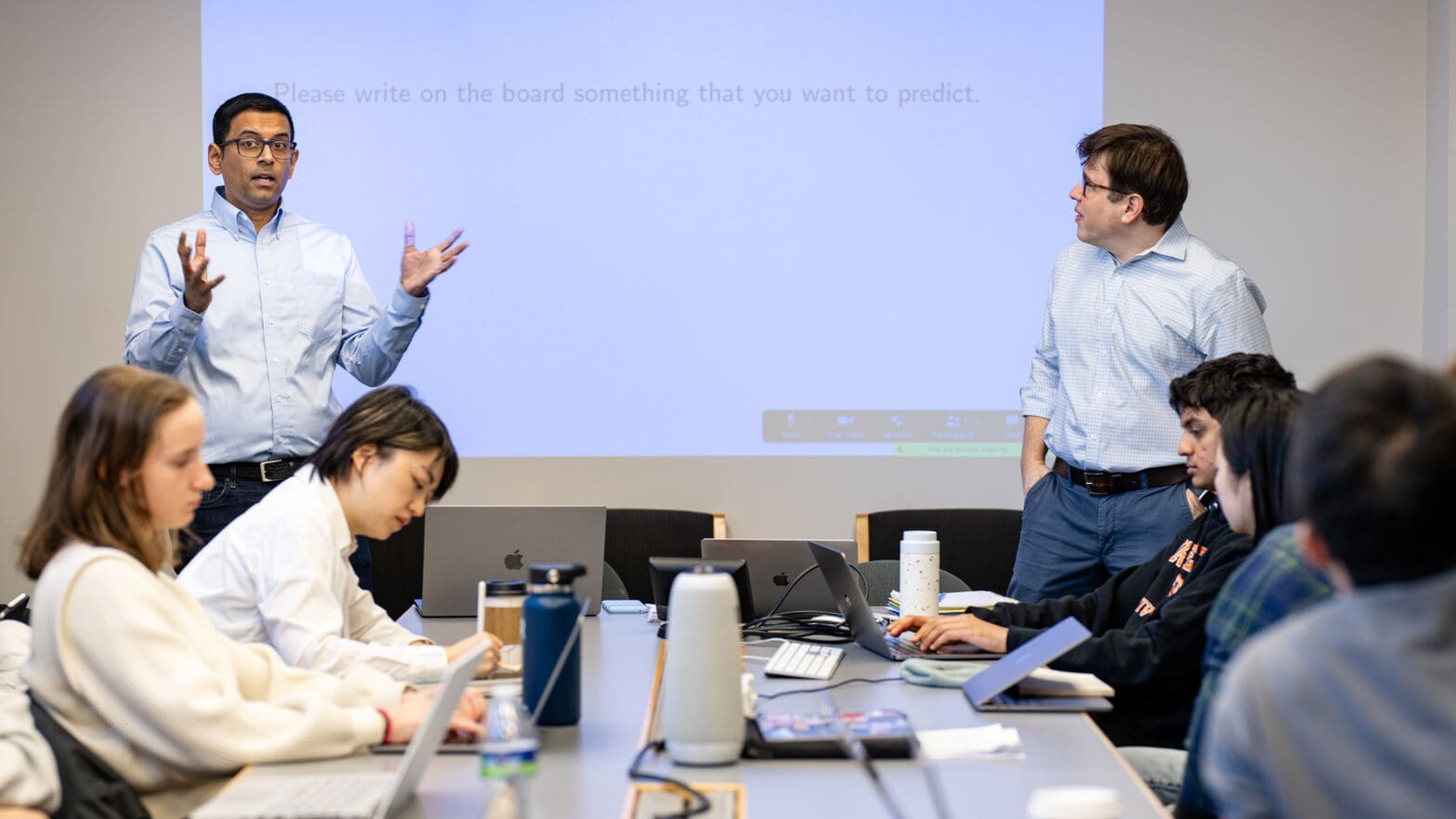
Is AI too dangerous to release openly?
By
on
The question of who should control AI development and who should have access to AI is of vital importance to society. Most leading models today (OpenAI’s GPT-4, Anthropic’s Claude 2, Google’s Gemini) are closed: They can only be used via interfaces provided by the developers. But many others, such as Meta’s Llama-2 and Mistral’s Mixtral, are open: Anyone can download them, run them, and customize them. Their capabilities are a step below the leading closed models because of a disparity in resources used to train them. For example, GPT-4 reportedly cost over $100 million, whereas Llama-2 required under $5 million. This is another reason Zuckerberg’s claims are interesting: He also announced that Meta is spending about $10 billion to acquire the computational resources needed to train AI. This means that the gulf in capabilities between open and closed models is likely to close or narrow.
Last fall, we collaborated with Stanford University to organize a virtual workshop to discuss the benefits and risks of openness in AI. We then assembled a team consisting of the organizers, many of the speakers, and a few collaborators to do an evidence review. What we found was surprising. Once we looked past the alarmism, we found very little evidence that openly released advanced AI, specifically large language models, could help bad actors more than closed ones (or even the non-AI tools available to them).
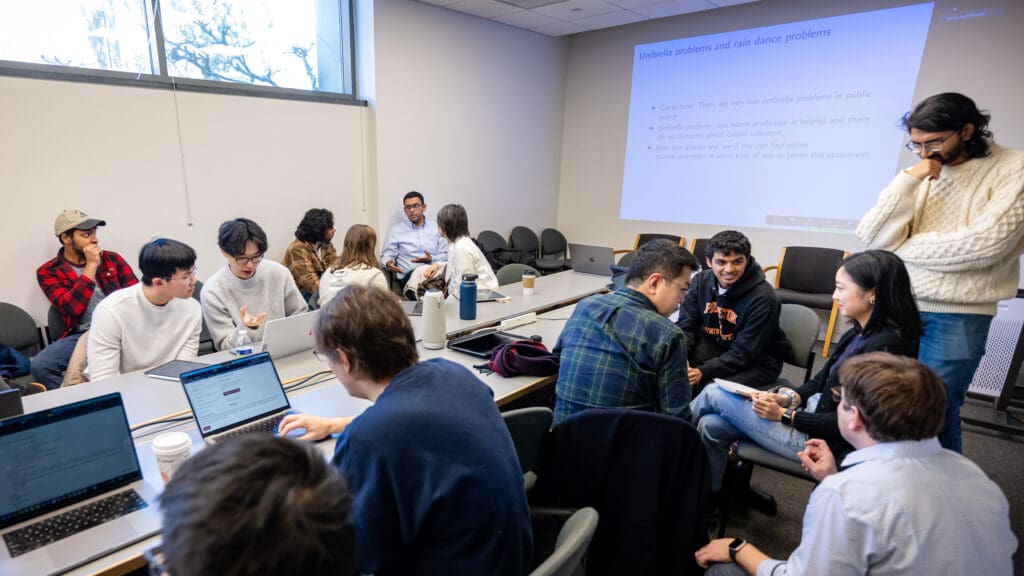
For example, a paper from MIT researchers claimed that AI could increase biosecurity risks. However, the information they found using open models was widely available on the internet, including on Wikipedia. In a follow-up study from RAND, a group of researchers in a simulated environment who had access to open models was no better at developing bioweapons than a control group that only had access to the internet. And when it comes to AI for cybersecurity, the evidence suggests that it helps defenders more than attackers.
We’ve noticed a pattern. Speculative, far-out risks such as bioterrorism, hacking nuclear infrastructure, or even AI killing off humanity generate headlines, make us fearful, and skew the policy debate. But the real dangers of AI are the harms that are already widespread. Training AI to avoid outputting toxic content relies on grueling work by low-paid human annotators who have to sift through problematic content, including hate speech and child sexual abuse material. AI has already led to labor displacement in professions such as translation, transcription, and creating art, after being trained using the creative work of these professionals without compensation. And lawyers have been sanctioned for including incorrect citations in legal briefs based on ChatGPT outputs, showing how overreliance on imperfect systems can go wrong.
Perhaps the biggest danger is the concentration of power in the hands of a few tech companies. Open models are in fact an antidote to this threat. They lower barriers to entry and promote competition. In addition, they have already enabled a vast amount of research on AI that could not be done without being able to download and examine the model’s internals. They also benefit research that uses AI to study other scientific questions, say in chemistry or social science. For such research, they enable reproducibility. In contrast, closed model developers often deprecate or remove access to their older models, which leads to research based on these models being impossible to reproduce.
So far, we’ve mainly talked about language models. In contrast, for image or voice generators, open models have already been shown to pose significant risk compared to closed ones. Offshoots of Stable Diffusion, a popular open text-to-image model, have been widely used to generate non-consensual intimate imagery (NCII), including of real people. While Microsoft’s closed model used to generate nude images of Taylor Swift was quickly fixed, such fixes are impossible to implement for open models — malicious users can remove guardrails because they have access to the model itself.
There are no easy solutions. Image generators have gotten extremely cheap to train and to run, which means there are too many potential malicious actors to police effectively. We think regulating the (mis)use of these models, such as cracking down on platforms used to distribute NCII, is much more justified and enforceable than regulating the development and release of the models themselves.
In short, we don’t think policymakers should be rushing to put AI back in the bottle, although the reasons for our recommendation are slightly different for language models versus other kinds of generative AI models. While we should be cautious about the societal impact of large AI models and continue to regularly re-evaluate the risks, panic around their open release is unwarranted. An open approach will also make it easier for academia, startups, and hobbyists to contribute to building and understanding AI. It is vital to ensure that AI serves the public interest. We should not let its direction be dictated by the incentives of big tech companies.
Arvind Narayanan is a professor of computer science and director of Princeton’s Center for Information Technology Policy (CITP). Sayash Kapoor is a computer science Ph.D. candidate at CITP; his research focuses on the societal impact of AI.
Narayanan and Kapoor are currently coauthoring a book, “AI Snake Oil,” which looks critically at what AI can and cannot do.
CITP is an initiative of Princeton University’s School of Engineering and Applied Science and the School of Public and International Affairs. The center’s researchers work to better understand and improve the relationship between technology and society.