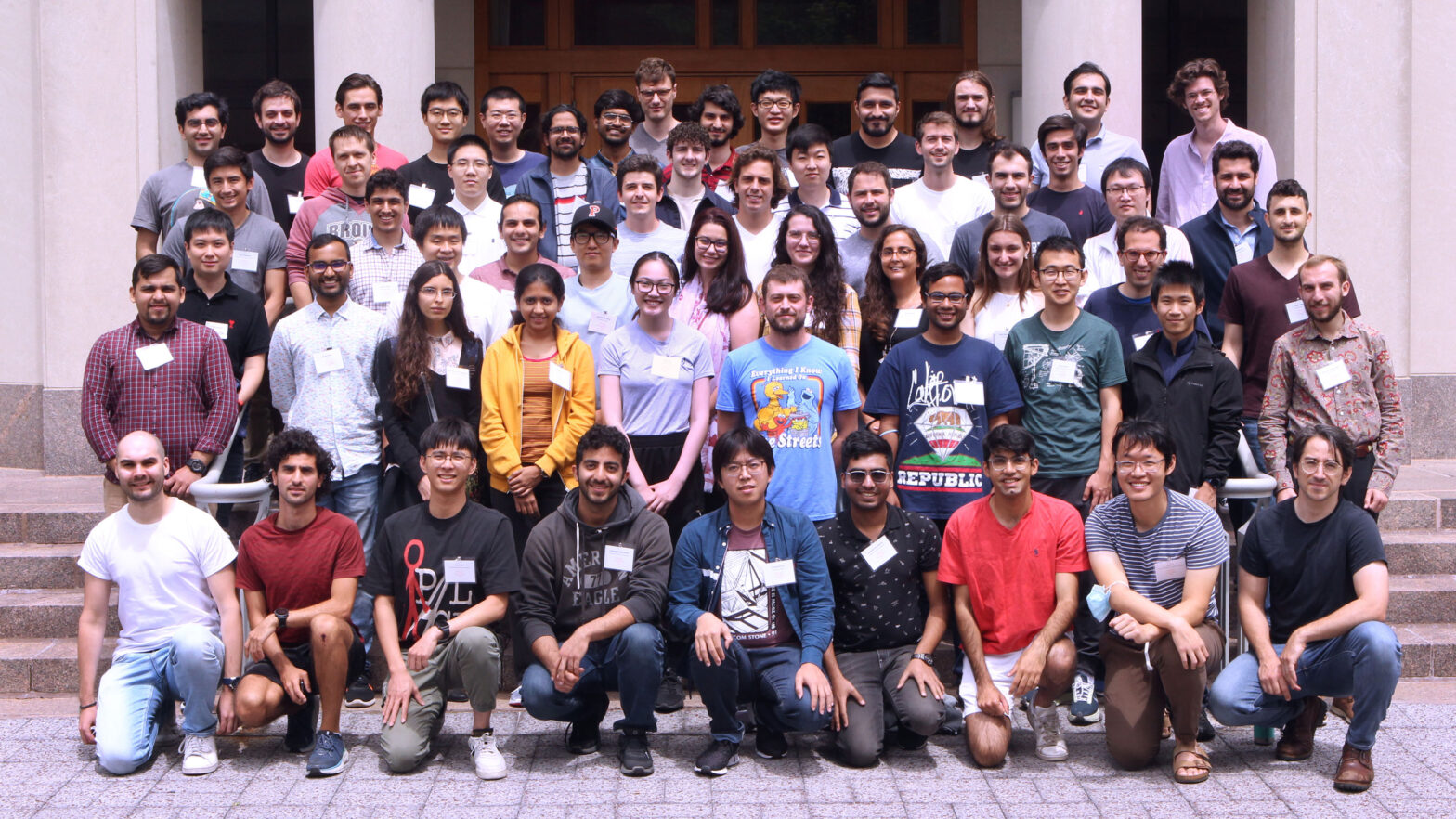
Machine Learning Theory Summer School fosters research community in a fast-growing field
Fundamental theories provide a framework to accelerate the pace of improvements in technology. This has been true for many technologies throughout history. For example, steam locomotives functioned for decades before scientists formalized underlying theories of statistical mechanics and thermodynamics — and used them to unlock technologies including racecars, reactors and rocket engines.
The development of machine learning — dominated by artificial neural networks that are roughly inspired by the structures of the human brain — has been far faster than that of the steam engine, but researchers still “lack a language to talk about why it is that modern machine learning works so well,” said Boris Hanin, an assistant professor of operations research and financial engineering (ORFE).
Last year, Hanin organized the first Princeton Machine Learning Theory Summer School to help promote a common language for the field, broadly defined as algorithms that use data to make predictions. With the dual goal of training the next generation of researchers, the first school launched virtually; four lecturers from different institutions spent a week teaching more than 200 graduate students from throughout the U.S. and other countries.
This year, the school convened in person on the Princeton campus from June 13 to 17. Sixty students came from more than 20 institutions in six countries to learn from academic and industry experts in machine learning theory. The students also shared their own work and ideas at a poster session and through impromptu discussions over meals and during breaks.
“I want to maximize the number of collision hours between these students,” said Hanin. “You work with people on problems in these classes, and they become your collaborators later — they become people you are associated with for life.”
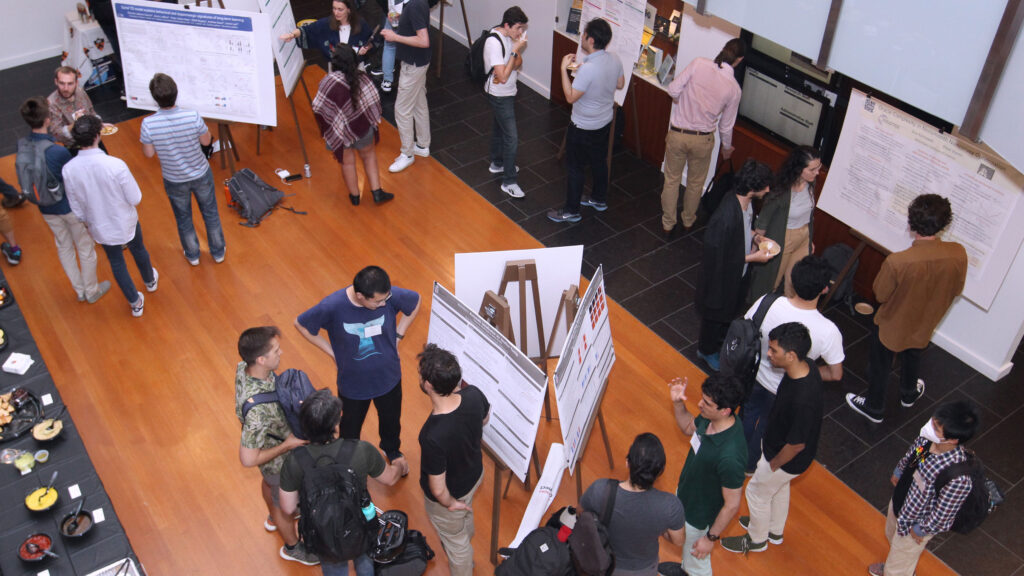
Hanin said he received a staggering number of applications for both years of the summer school, reflecting that “there appear to be very few systematic educational opportunities for young people to get into the field” and to learn to identify important research questions in a rapidly growing area.
One challenge, he said, is that many of the senior researchers in deep learning theory for neural networks are still early in their careers, meaning that opportunities for graduate students to learn from experts in the field are limited.
The backgrounds of this year’s participants reflect both the broadening influence of machine learning and the growing interest of students from different disciplines: Two lecturers, Nati Srebro of the Toyota Technical Institute of Chicago and Tengyu Ma of Stanford University (who did his Ph.D. work with Princeton professor Sanjeev Arora), come from computer science backgrounds, while Soledad Villar of Johns Hopkins University and Sébastien Bubeck of Microsoft Research (a former Princeton ORFE faculty member) are mathematicians. Graduate student participants came from computer science and applied mathematics backgrounds, as well as fields including statistics, electrical engineering, neuroscience and physics.
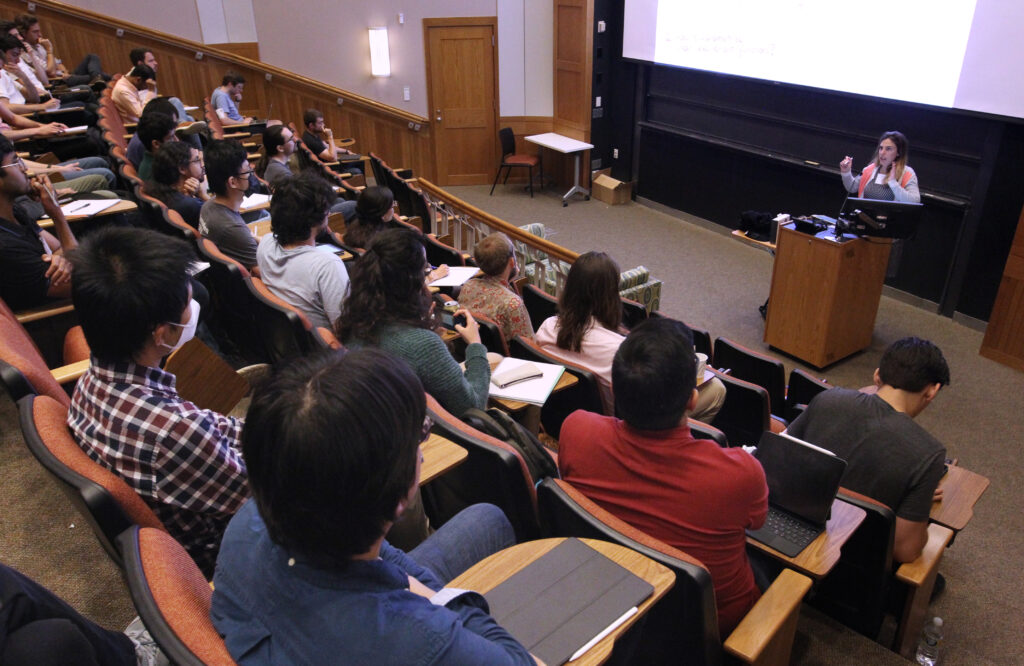
Several students said they had worked as software developers or studied other aspects of computer science, but became curious about the workings of the machine learning algorithms they were using, spurring them to pivot to machine learning theory.
Noam Razin, a rising third-year doctoral student at Tel Aviv University, said the Princeton summer school was the first time he had gathered with a community of machine learning researchers outside his institution — a critical opportunity for sharing ideas and broadening his perspective on the fast-moving field.
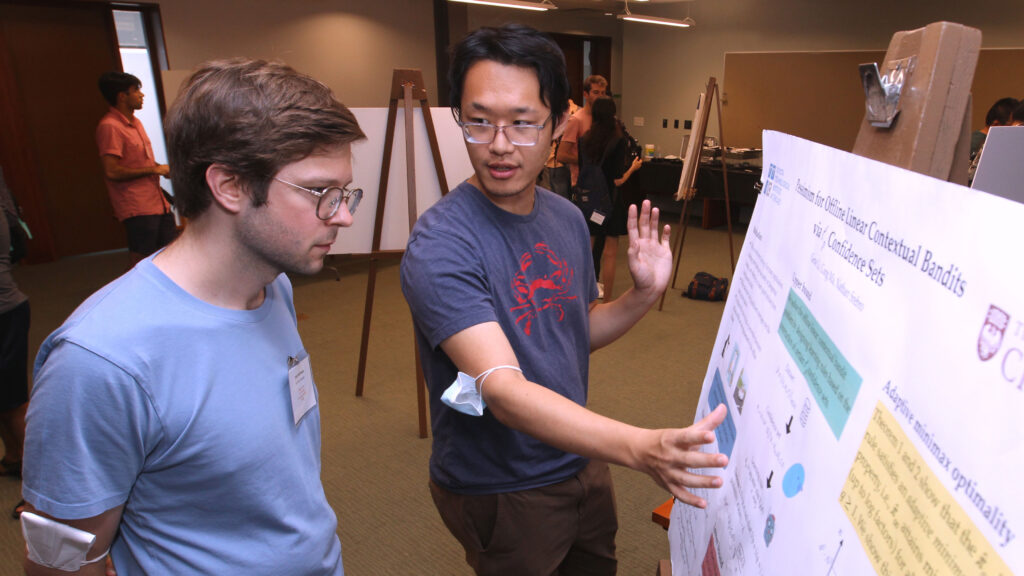
Tianyu He, a physics Ph.D. student from Brown University, said he met students who were using different approaches to solve problems similar to those in his own work, which he found inspiring — in addition to learning from lecturers at the forefront of the field.
Since his arrival at Princeton in 2020, Hanin said he’s been impressed by the University’s high level of support and enthusiasm for educational endeavors like the Machine Learning Theory Summer School. The school is supported in part by Hanin’s CAREER grant from the National Science Foundation, in addition to Princeton’s Department of Operations Research and Financial Engineering, Center for Statistics and Machine Learning, School of Engineering and Applied Science, and Program in Applied and Computational Mathematics.