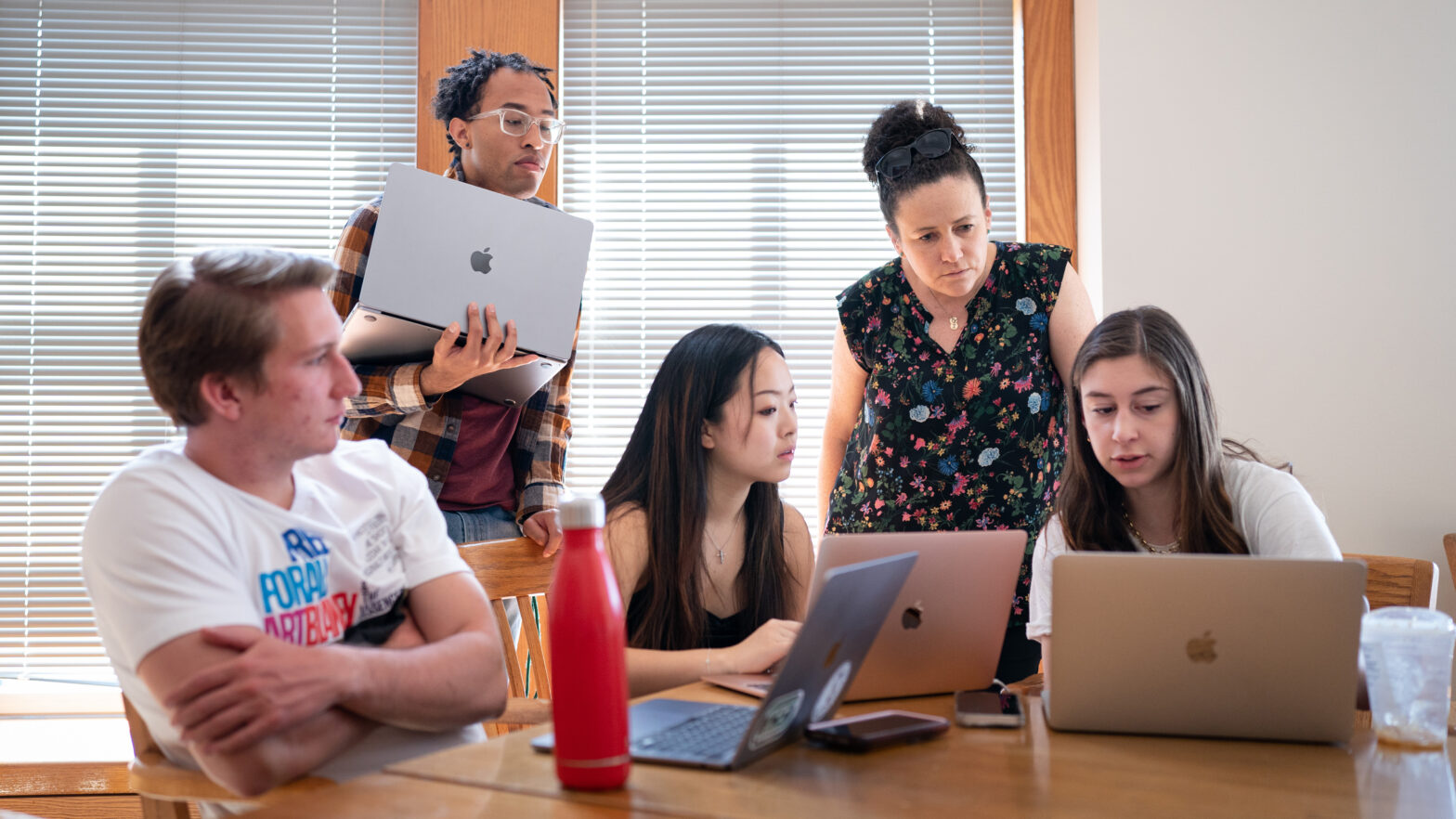
Using the tools of computer science to improve health care
By
on
“Medicine is becoming more and more a data-driven enterprise,” said Olga Troyanskaya, the seminar instructor. Troyanskaya, a professor of computer science and the Lewis-Sigler Institute for Integrative Genomics, is the director of a new University initiative, Princeton Precision Health. Integrating computer science, biology, social science, policy, psychology, ethics and medicine, the initiative uses large, complex data sets to make health care policy and delivery more precise, effective and unbiased.
Created in the summer of 2022, the project focuses on three areas: kidney disease and diabetes, the immune system and inflammation, and neurodevelopment and mental health. In each, Troyanskaya said, “there is enormous unmet need to develop precision medicine approaches and correct existing biases.” In the case of chronic kidney disease, Princeton Precision Health is collaborating with large initiatives that generate data at the genetic, molecular, socioeconomic and clinical levels. There are also well-documented racial disparities in the incidence of chronic kidney disease.
Princeton Precision Health’s goal is to take a comprehensive, data-driven approach to this type of health care challenge. Currently, researchers’ use of data is limited to the approaches specific to their discipline. Social scientists rarely include comprehensive genetic information in their work, and computational biologists rarely use social science data. Instead of working in silos, the initiative aims to bring experts from many fields together to collaborate on interdisciplinary data sets created with cutting-edge tools. Importantly, Troyanskaya said, ethical frameworks and behavioral modeling are integral to Princeton Precision Health’s approach to achieve effective and unbiased clinical outcomes and health policies.
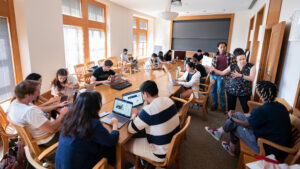
For example, in applying this approach to the study of kidney disease, Troyanskaya said, it’s possible that the initiative’s data scientists could develop a machine learning approach for integrative genetic analysis to identify a predictor for transplant success. Bioethicists and policy experts could then use that data to create fairer and more equitable transplant policies.
David Kinney, an analytic philosopher and expert in formal modeling, is working with Princeton Precision Health precisely to lend this type of interdisciplinary depth. He plans to use data from large kidney cohort studies to investigate the ethics of using genetic markers in treatment protocols. It’s difficult to determine, he said, when a genetic marker is just a proxy for sex or race and when it has a causal impact on disease development or treatment. “Using these really rich datasets to show what kinds of fair inferences we can and cannot make is really an exciting project,” he said.
“This is exactly the kind of interdisciplinary collaboration we need to address issues of health, data and policy,” said Betsy Levy Paluck, professor of psychology and public affairs at Princeton and a member of the initiative’s executive committee.
While it may seem unusual to create a precision health initiative outside of a medical center, the establishment of Princeton Precision Health has distinct advantages, according to Troyanskaya. Academic researchers take a broad, longer-term, highly interdisciplinary view of these complex problems. “We can ask, and potentially answer, very hard questions involving researchers across domains, from molecular biology to social science, psychology and philosophy,” Troyanskaya said.
Troyanskaya’s work on the initiative spurred her to offer the University’s first undergraduate course in computational methods in precision health. Somewhat to her surprise, the class quickly filled and even attracted a waiting list. No background in biology or medicine is required. Troyanskaya said students are researching topics including microscopic anatomy, access to abortion care and racial bias in cancer treatment.
All 19 students in the class are computer science concentrators fulfilling the department’s requirement for independent research. Aria Nagai, a junior, said that what drew her to this topic was its focus on using computer science tools to address real-world problems. “I’m not super interested in the theory behind algorithms, or something like that,” she said. “I’m more interested in learning how to use algorithms or machine learning to help others.” That interest is shared with her research collaborators in the seminar, Hervé Ishimwe and Louis Pang, also juniors.
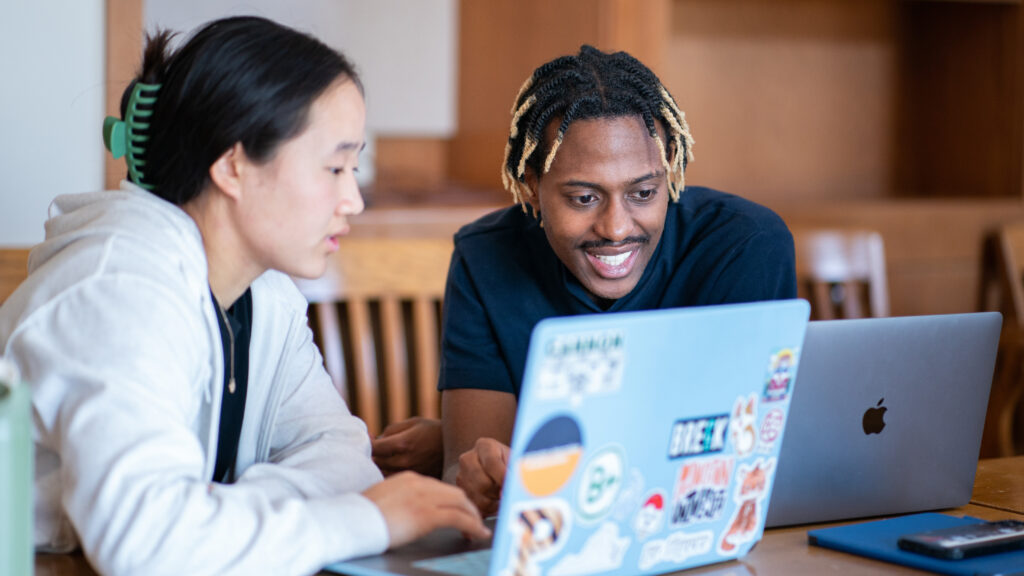
Of the three, only Ishimwe has a longstanding interest in medicine and public health. Pang has worked for affordable housing nonprofits; Nagai has done internships in K-12 education. “An interest and passion for public service,” Nagai said, spurred her to take the class. “Data can bring a lot of things to light” in public policy, she said, including funding concerns and gaps in social services. Nagai and her collaborators see the class as a unique opportunity to practice applying computer science tools to questions of public policy and public service.
Their research project is focused on how urban diversity affects health outcomes for minority populations in New York City. Each is studying a different health outcome: Nagai is looking at low birthweight, Ishimwe at cancer, and Pang is focused on violent deaths and drug overdoses. They hope to create visual representations of the key factors that impact these health outcomes according to the data. The group’s goal, Ishimwe said, is to create tools that can help people quickly understand what factors, like distance to a hospital or paid sick leave, are influencing certain health outcomes. “We want to try and help policymakers make practical decisions,” he said.
Finding and coding the data they needed was a major challenge, and they ultimately had to modify their initial project scope because of some limitations in the available data. One of the biggest lessons he’s taken from the class, Pang said, is learning when data should not be used. Troyanskaya and Tavis Reed, a graduate student in the Quantitative and Computational Biology program and assistant instructor in the course, have been careful to illustrate cases where making conclusions based on the available data might be unwise or even unethical. Learning the limits of data has been as eye-opening as learning about its uses, Pang said.
In the coming months the initiative will be launching research projects, funding research proposals, hiring postdoctoral researchers and data scientists and hosting workshops and seminars. Troyanskaya will be giving a talk at Princeton Reunions on Friday, May 26, at 3 p.m. in Icahn 101 for alumni interested in the work of the initiative. The initiative will also host a full-day conference on precision health on September 29, 2023, at the Friend Center.
Interested in future Princeton Precision Health news, events and funding opportunities? Visit the Princeton Precision Health website.